InfluxDB for IoT Monitoring Data Platform for Digital Transformation
The enterprise IoT solution using InfluxDB provides real-time insights and analytics into devices and processes—collecting, storing, and visualizing sensor data.
#1
Time Series Database
Source: DB Engines
1B+
Downloads of InfluxDB
Take the complexity out of time series data management
Try InfluxDBTurn high-volume, high-velocity, high-resolution time series data into actionable intelligence without sacrificing performance.
Get high-speed ingestion, real-time querying, and seamless scalability in a highly performant time series database that can handle unlimited volumes of time series data, even as data complexity and cardinality increases.
Run InfluxDB at scale in any environment in the cloud, on-premises, or at the edge. InfluxDB consists of the core database and storage engine, an API, and an ecosystem of tools and integrations to manage time-stamped data. Easily integrate with 5K+ prebuilt connections using Telegraf, our open source agent with 5B+ downloads.
Run InfluxDB 3 where you need it
Start for free, scale in seconds
Start NowFor small workloads and getting started
Deploy anywhere: on-prem, private cloud, edge, or multi-tenant cloud.
Secure, dedicated cloud infrastructure
Run a Proof of ConceptFor scaled workloads
Fully-managed, single-tenant service with unlimited scale, high availability, secure private connections, and enhanced Support.
Own your data with enterprise features
Run a Proof of ConceptFor scaled workloads
Control your infrastructure with unlimited scale, high availability, enterprise-grade security, and enhanced Support. On-prem, private cloud, and edge deployment.
Why InfluxDB
Limitless data collection
Ingest and analyze millions of time series data points per second without limits or caps.
Predict and prevent
Maximize uptime and prevent costly failures with real-time monitoring and preventative insights.
Real-time query
Data is immediately available for querying in InfluxDB, enabling real-time SQL queries and lightning-fast responses.
Why a purpose-built time series database?
Sensor data is time-stamped to help you understand how your processes and equipment are performing over time, facilitating further innovation and improvement.
Why InfluxDB for Enterprise IoT?
Nearly 60 billion connected IoT devices are predicted to be in use by 2025. This data is streaming in real time, motivating companies to identify an IoT data platform architecture that is resilient, scalable, and extensible enough to handle these new workloads.
Built for developers, InfluxDB is central to many IoT solutions, providing high throughput data ingestion, compression, and real-time querying. Efficiency and effectiveness must start in the data structure, ensuring that time-stamped values are collected with the necessary precision and metadata to provide flexibility and speed in graphing, querying, and alerting.
Using historical data from sensors to gain actionable insights creates a major competitive advantage. Predictive maintenance, optimized traffic routing, reduced churn management, and enhanced water conservation are all possible with IoT analytics.
With the speed and velocity of events generated by sensors, businesses want to act on data in real time without human interaction. For example, automatically shutting down a pump if a leak is detected or changing turbine direction based on forecasted wind speed creates an immediate business advantage.
Related resources for developers:
Get InfluxDB
InfluxDB Integrations
Free InfluxDB training
“InfluxDB is helping Bboxx become a data-driven business. Instead of just selling boxes, we’ll soon be selling customers a pay-by-the-month plan. It’s the speed, reliability, and scalability of InfluxDB that enables us to move ahead with this more flexible and responsive plan.”
David McLean
Senior Developer, Bboxx
Open data standards drive performance and interoperability
InfluxDB 3 is built in Rust and the FDAP stack—Flight, DataFusion, Arrow, and Parquet—leveraging Apache-backed technologies to efficiently ingest, store, and analyze time series data at any scale.
Get InfluxDBF
Flight for efficient columnar data transfer
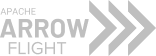
D
DataFusion for high-performance querying
A
Arrow for optimized in-memory columnar analytics
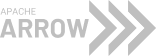
P
Parquet for high-compression storage
InfluxDB’s columnar database supports time series data at a lower TCO
See ways to get started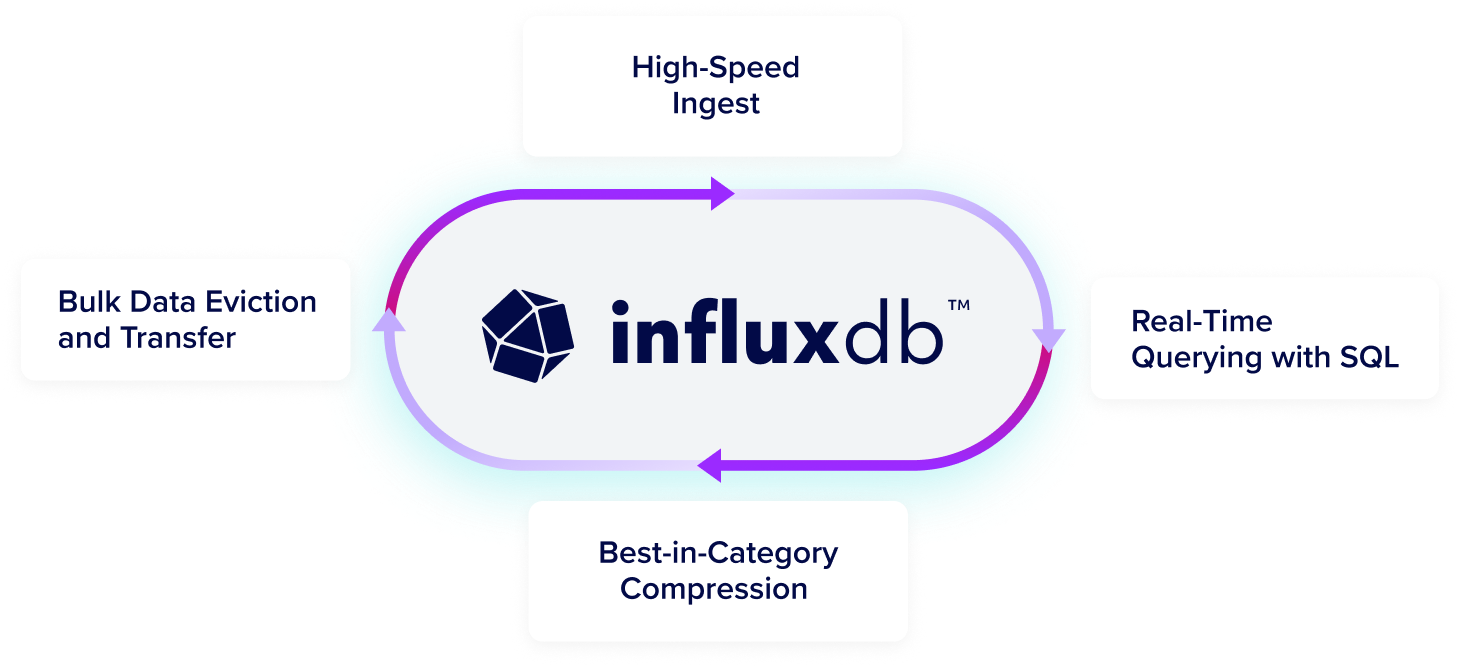
Loved by developers, trusted by enterprises
65M+
daly events processed
45x
more resource efficient
Siemens Energy
“[We] long used InfluxDB open source... Moving to commercial InfluxDB was a strategic move to unify our data infrastructure, ensuring we have the reliability, scalability, and real-time performance to keep pace with production... and proactive maintenance.”
Jan Petersen
Senior Manufacturing Engineer
500M+
Metrics collected daily
Mission-critical monitoring
Real-time data access for queries
LOFT ORBITAL
Space Made Simple: How Loft Orbital Delivers Unparalleled Speed-to-Space with InfluxDB Cloud
Read Case Study65M+
daly events processed
45x
more resource efficient
CAPITAL ONE
"InfluxDB is a high-speed read and write database. The data is written in real-time, you can read it in real-time, and while reading, you can apply your machine learning model. So, in real-time, you can forecast and detect anomalies."
Rajeev Tomer
Sr. Manager of Data Engineering
50%
lower total cost of ownership
100K
real-time metrics with simplified deployment
TERÉGA
Teréga Replaced Its Legacy Data Historian with InfluxDB
Lorem ipsum
Lorem ipsum dolor sit amet consectetur.
65M+
daly events processed
45x
more resource efficient
WIDEOPENWEST
"I was blown away with how easy it was to install and configure InfluxDB. The clustering was easy. The documentation was great, and the support has been second to none."
Dylan Shorter
Engineer III, Software and Product Integration Engineering
45%
Less equipment downtime
10%
Reduced waste
MAJIK SYSTEMS
From Reactive to Proactive: How MAJiK Systems Embraced Predictive Maintenance with InfluxDB and Time Series Data
Dylan Shorter
Engineer III, Software and Product Integration Engineering
65M+
daly events processed
45x
more resource efficient
JU:NIZ ENERGY
“With InfluxDB Cloud Dedicated, the great thing is that we don't need to think about data storage costs or usage anymore because data storage gets way cheaper.”
Ricardo Kissinger
Head of IT Infrastructure and IT Security