Time series database explained
In this technical paper, InfluxData CTO, Paul Dix, will walk you through what time series is (and isn’t), what makes it different from stream processing, full-text search and other solutions.
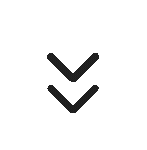
By reading this tech paper, you will:
- Learn how time series data is all around us,
- See why a purpose built TSDB is important.
- Read about how a Time Series database is optimized for time-stamped data.
- Understand the differences between metrics, events, & traces and some of the key characteristics of time series data.
What is a time series database?
A time series database (TSDB) is a database optimized for time-stamped or time series data. Time series data are simply measurements or events that are tracked, monitored, downsampled, and aggregated over time. This could be server metrics, application performance monitoring, network data, sensor data, events, clicks, trades in a market, and many other types of analytics data.
A time series database is built specifically for handling metrics and events or measurements that are time-stamped. A TSDB is optimized for measuring change over time. Properties that make time series data very different than other data workloads are data lifecycle management, summarization, and large range scans of many records.
Why is a time series database important now?
Time series databases are not new, but the first-generation time series databases were primarily focused on looking at financial data, the volatility of stock trading, and systems built to solve trading. But financial data is hardly the only application of time series data anymore — in fact, it’s only one among numerous applications across various industries. The fundamental conditions of computing have changed dramatically over the last decade. Everything has become compartmentalized. Monolithic mainframes have vanished, replaced by serverless servers, microservers, and containers.
Today, everything that can be a component is a component. In addition, we are witnessing the instrumentation of every available surface in the material world — streets, cars, factories, power grids, ice caps, satellites, clothing, phones, microwaves, milk containers, planets, human bodies. Everything has, or will have, a sensor. So now, everything inside and outside the company is emitting a relentless stream of metrics and events or time series data.
Want to know more?
Download the PaperThis means that the underlying platforms need to evolve to support these new workloads — more data points, more data sources, more monitoring, more controls. What we’re witnessing, and what the times demand, is a paradigmatic shift in how we approach our data infrastructure and how we approach building, monitoring, controlling, and managing systems. What we need is a performant, scalable, purpose-built time series database.
What distinguishes the time series workload?
Time series databases have key architectural design properties that make them very different from other databases. These include time-stamp data storage and compression, data lifecycle management, data summarization, ability to handle large time series dependent scans of many records, and time series aware queries.
For example: With a time series database, it is common to request a summary of data over a large time period. This requires going over a range of data points to perform some computation like a percentile increase this month of a metric over the same period in the last six months, summarized by month. This kind of workload is very difficult to optimize for with a distributed key value store. TSDB’s are optimized for exactly this use case giving millisecond level query times over months of data. Another example: With time series databases, it’s common to keep high precision data around for a short period of time. This data is aggregated and downsampled into longer term trend data. This means that for every data point that goes into the database, it will have to be deleted after its period of time is up. This kind of data lifecycle management is difficult for application developers to implement on top of regular databases. They must devise schemes for cheaply evicting large sets of data and constantly summarizing that data at scale. With a time series database, this functionality is provided out of the box.
Independent ranking of top 15 time series databases
Time series databases are the fastest growing segment in the database industry. But which time series database is the best and most popular? There are many ways of determining popularity, but an independent website, DB-Engines, ranks databases based on search engine popularity, social media mentions, job postings, and technical discussion volume. (Read their full methodology). Here are the current results:
To see trends over time, the following graphic shows the top 10 time series databases and their historical changes:
Time series – the fastest growing database category
DB-Engines also ranks time series database management systems (Time Series DBMS) according to their popularity. Time series databases are the fastest growing segment of the database industry over the past year.
What makes InfluxDB time series database unique?
InfluxDB was built from the ground up to be a purpose-built time series database; i.e., it was not repurposed to be time series. Time was built-in from the beginning. InfluxDB is part of a comprehensive platform that supports the collection, storage, monitoring, visualization and alerting of time series data. It’s much more than just a time series database.
The whole InfluxData platform is built from an open source db core. InfluxData is an active contributor to the Telegraf, InfluxDB, Chronograf and Kapacitor (TICK) projects — the “I,C,K” from the TICK Stack is being collapsed into a single binary in InfluxDB 2.0 — as well as selling InfluxDB Enterprise and InfluxDB Cloud on this open source core. The InfluxDB data model is quite different from other time series solutions like Graphite, RRD, or OpenTSDB. InfluxDB has a line protocol for sending time series data which takes the following form: measurement-name tag-set field-set timestamp
. The measurement name is a string, the tag set is a collection of key/value pairs where all values are strings, and the field set is a collection of key/value pairs where the values can be int64, float64, bool, or string. The measurement name and tag sets are kept in an inverted index which make lookups for specific series very fast. For example, if we have CPU metrics:
cpu,host=serverA,region=uswest idle=23,user=42,system=12 1464623548s
Timestamps in InfluxDB can be second, millisecond, microsecond, or nanosecond precision. The micro and nanosecond scales make InfluxDB a good choice for use cases in finance and scientific computing where other solutions would be excluded. Compression is variable depending on the level of precision the user needs. On disk, the data is organized in a columnar style format where contiguous blocks of time are set for the measurement, tagset, field. So, each field is organized sequentially on disk for blocks of time, which make calculating aggregates on a single field a very fast operation. There is no limit to the number of tags and fields that can be used.
Other time series solutions don’t support multiple fields, which can make their network protocols bloated when transmitting data with shared tag sets. Most other time series solutions only support float64 values, which means the user is unable to encode additional metadata along with the time series. Even OpenTSDB and KairosDB, which support tags (unlike Graphite and RRD), have limitations on the number of tags that can be used. At around 5 to 6 tags, the user will start seeing hot spots within their cluster of HBase or Cassandra machines.
InfluxDB doesn’t have this limitation because the InfluxDB data model is designed for time series specifically. It pushes the developer in the right direction to get good performance out of the database by indexing tags and keeping fields unindexed. It’s flexible in that many data types are supported, and the user can have many fields and tags. Because of all these factors, a purpose-built time series database like InfluxDB is the best solution for working with time series data.
Time series database use cases
Time series databases have become essential infrastructure for organizations dealing with continuous streams of timestamped data. Their specialized architecture makes them ideal for scenarios where traditional databases struggle with performance and scalability. Let’s explore the most common use cases where time series databases truly shine.
Infrastructure and Application Monitoring
One of the most widespread applications of time series databases is monitoring IT infrastructure and applications. Organizations use them to track server metrics like CPU usage, memory consumption, disk I/O, and network traffic. These databases excel at ingesting millions of data points per second from thousands of servers, containers, and microservices while maintaining sub-second query performance.
DevOps teams rely on this capability to detect anomalies, set up intelligent alerting systems, and perform root cause analysis when issues arise. The ability to quickly aggregate data in time ranges from nanoseconds to months enables both real-time troubleshooting and long-term capacity planning.
IoT
The Internet of Things generates massive volumes of time series data from sensors measuring everything from temperature and humidity to vibration and pressure. Time series databases provide the backbone for smart cities tracking traffic patterns, manufacturing plants monitoring equipment health, and agricultural systems optimizing irrigation based on soil moisture levels.
These databases handle the unique challenges of IoT data: high-frequency updates, out-of-order data arrival, and the need to store raw data alongside aggregated summaries. They enable organizations to build predictive maintenance systems that can forecast equipment failures before they occur, potentially saving millions in downtime costs.
Financial Markets and Trading
Financial institutions use time series databases to store and analyze market data, including stock prices, trading volumes, and order book information. The ability to process millions of trades per second while maintaining data accuracy is crucial for algorithmic trading systems, risk management platforms, and regulatory compliance.
These databases support complex queries needed for technical analysis, such as calculating moving averages, identifying price patterns, and backtesting trading strategies across years of historical data. The combination of high write throughput and fast analytical queries makes them indispensable for modern financial technology.
Energy and Utilities Management
Power grids, water systems, and renewable energy installations generate continuous streams of operational data. Time series databases help utilities companies monitor grid stability, predict demand patterns, and optimize resource distribution. Smart meter deployments, which can generate readings every few minutes from millions of homes, rely heavily on these specialized databases.
Energy companies use this data to balance supply and demand in real-time, integrate renewable sources more effectively, and provide customers with detailed usage insights. The ability to perform complex aggregations across geographical regions and time periods enables better grid management and more accurate billing.
Business Analytics and User Behavior
Companies track numerous time-based metrics to understand their business performance and user behavior. Website analytics, application performance metrics, sales data, and customer engagement statistics all naturally fit the time series model. These databases enable businesses to track key performance indicators in real-time, identify trends, and make data-driven decisions.
E-commerce platforms use time series databases to monitor conversion rates, track inventory levels, and analyze seasonal patterns. The ability to quickly compare current performance against historical baselines helps businesses respond rapidly to changing market conditions.
Time series databases: FAQ
Listed below for quick reference are brief answers to frequently asked questions about time series databases:
What is a time series database?
Here’s a brief time series database definition: A time series database (TSDB) is a database optimized for time-stamped (time series) data and for measuring change over time.
What is the best time series database?
Visit this page to learn about what makes a powerful time series database and which database is best for storing large volumes of time series data.
What are time series data examples?
Visit the What is time series data page to view time series data examples.
Is InfluxDB open source?
InfluxDB is an open source time series database with a large and vibrant community.
Can I use InfluxDB with Grafana?
There are thousands of use cases utilizing InfluxDB and Grafana. Visit our Community Showcase to read about them.
How does InfluxDB compare to other databases?
View InfluxDB benchmarking tests comparing its performance to other databases (such as Cassandra, Elasticsearch, MongoDB, OpenTSDB, Graphite and Splunk) based on parameters such as write throughout, query throughput, and on-disk storage.
Is a time series database better than a relational database for handling time series data?
If you’re debating time series database vs relational database, a time series database (TSDB) is specific for sorting and querying time series data, and tends to be more efficient than a relational database, which is more generic.
Can I use a time series database for edge computing?
Transmitting data from the edge to cloud in a reliable way continues to be a challenge for many businesses. Read the Edge Computing & Data Replication with InfluxDB e-book to learn what ‘the edge’ is, edge computing use cases and benefits, and how InfluxDB time series database can be used for edge computing.
What is the difference between a time series database and a data warehouse?
While a time series database is a database optimized for time-stamped or time series data, a data warehouse stores and organizes data from multiple sources in a central location.