Predictive Maintenance in Industrial Operations
Collect and analyze large datasets in real-time to optimize maintenance, reduce downtime, and improve operational efficiency
Get Started Now
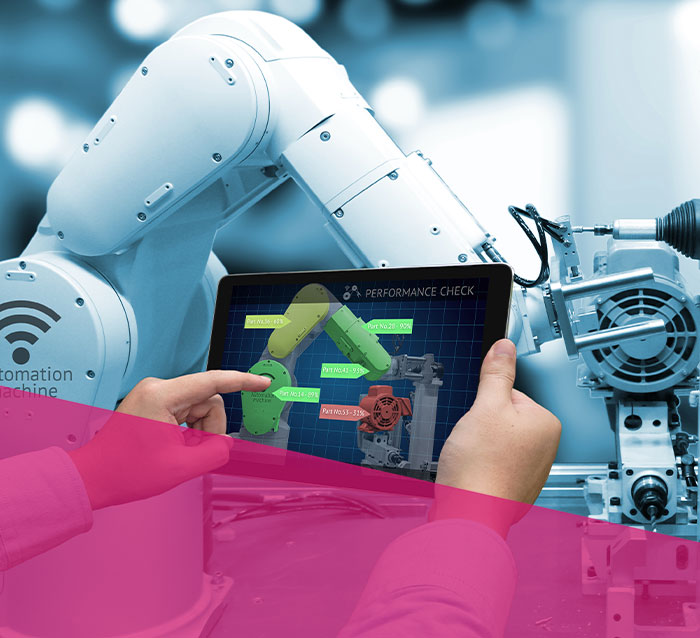
Why InfluxDB for industrial operations?
InfluxDB can handle the scale, volume, and precision of industrial data. It integrates with key Operations Technology stacks and ecosystems.
Why predictive maintenance?
A broken process
The established approach to industrial maintenance is reactionary and broken. Servicing industrial equipment occurs on a set schedule, or after it fails. In a sector where efficiency matters, this process is inefficient and expensive. It results in unnecessary maintenance on machines that don’t need it, or emergency repairs and unplanned downtime. All this costs money and negatively impacts your bottom line.
Improving effectiveness
Predictive maintenance is an approach that combines historical and real-time data with machine learning and advanced analytics tools to identify patterns and predict machine behavior.
For example, you can use historical data to understand the typical lifespan of a machine valve, and combine that with real-time data about the valve’s performance. When the valve starts to show signs of wear, you can schedule maintenance to replace the valve that minimizes the impact on production.
Powering predictive maintenance with InfluxDB
The reason predictive maintenance isn’t more widely practiced is because legacy data historians lack the necessary capabilities. Industrial data can originate from many different sources, arriving in different formats, and at different intervals. This can lead to data gaps with legacy data historians where industrial operators must make critical decisions with incomplete data.
InfluxDB is a purpose-built time series database designed to handle the time series workloads required for predictive maintenance. Providing business-critical insights faster allows industrial operators to set more accurate tolerances and indicators for when components need service or replacement. This improves failure predictions and generates more granular and timely alerts.
Architecture
Predictive maintenance processes rely on machine learning and advanced analytics tools. Training these tools requires massive amounts of data. Therefore, organizations need a way to collect, store, and manage all this data. They also need a solution that integrates with machine learning, analytics, and other tools needed to optimize predictive maintenance processes.
Telegraf is InfluxData’s open source, data collection agent. With 300+ plugins, it collects data from any system or protocol, including MQTT, ModBus, OPC-UA, Kafka, Node-Red, and more. Telegraf works seamlessly with InfluxDB, which provides equal access to ‘hot’, in-memory data, and ‘cold’ data in object storage. This means that organizations can access historical and real-time data with a single solution. When it comes to querying this data, InfluxDB integrates with visualization tools, like Grafana, to ensure stakeholders across the organization have the information they need at their fingertips.
Interoperability
Built using the open source Apache Arrow project, industrial organizations can extend and integrate InfluxDB with a wide range of tools, solutions, and ecosystems. InfluxDB saves data in the Apache Parquet file format, which many other ecosystems use (e.g., Hadoop, Snowflake, Cloudera). These solutions can read Parquet files directly, extending the value of your time series data.
What to talk with an expert?
Featured customers:
Related resources for developers
Get InfluxDB
Industry 4.0 and InfluxDB
InfluxDB Integrations
IIoT/OT Technical Paper
InfluxDB Edge Data Replication
Free InfluxDB training