Infrastructure Monitoring with InfluxDB | Live Demonstration
Anomaly Detection
Anomaly detection is the process of finding data points that are outliers from the rest of a data set.
What is Anomaly Detection?
Anomalies are data points that are greatly different from the rest of the data set they’re a part of. Data scientists may want to identify anomalies to investigate what’s causing them or to remove them from calculations they can misleadingly affect, such as means or standard deviations. Anomalies can be caused by instrument or measurement errors or they can be valid data points that simply differ greatly from what’s expected. In either case, identifying anomalies is the first step to understanding them.
How to detect anomalies?
One way of detecting anomalies is to set thresholds beyond which data is classified as an anomaly. A common way of setting thresholds is to use multiples of the standard deviation of a data set. If a data set has a normal distribution, 99.7% of data points will be within three standard deviations from the mean value. Statistical theory forms the basis of some common anomaly detection methods like z-scores and Grubb’s test. Other anomaly detection methods use density-based techniques, correlation-based detection, or neural networks. New methods of detecting anomalies are still being theorized, and different methods are more successful with different kinds of data sets.
Take charge of your operations and lower storage costs by 90%
Get Started for Free Run a Proof of ConceptNo credit card required.
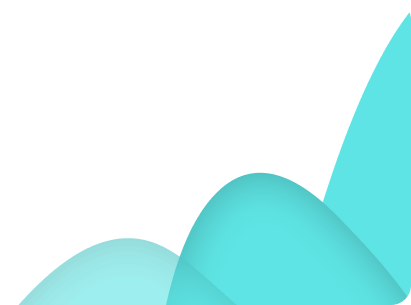