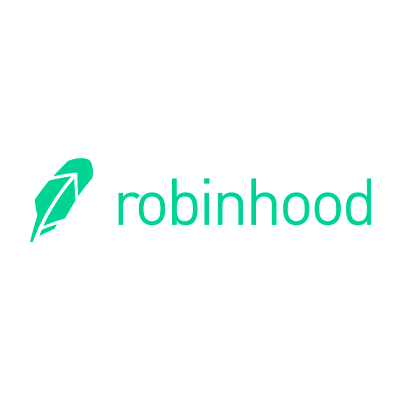
Robinhood’s story began at Stanford, where co-founders Baiju and Vlad were roommates and classmates. After graduating, they packed their bags for New York City and built two finance companies, selling their own trading software to hedge funds. There, they discovered that big Wall Street firms were paying next-to-nothing to trade stocks, while most Americans were charged commission for every single trade. So they decided to change that, and headed back to California to build a financial product that would enable everyone — not just the wealthy — access to financial markets.
A pioneer of commission-free investing, Robinhood is on a mission to democratize finance for all and believes the financial system should be built to work for everyone. Robinhood is democratizing the financial systems by offering commission-free investing and trading with the use of your phone or desktop. As exciting as that sounds to the outside world, internally, the team at Robinhood had to understand the different risk vectors and build engineering solutions to mitigate these risks. To build a real-time risk monitoring system, Robinhood chose InfluxDB and Faust, an open-source Python stream processing library for Kafka streams.
InfluxDays Presentation
Robinhood presented at InfluxDays San Francisco 2019. In this talk, they provided an overview of their real-time anomaly detection system which is used to monitor and mitigate risk. Additionally, they highlight Robinhood’s architecture, which is instrumental for time series anomaly detection and real-time stream processing.
Improved alerting
Automated threshold-based anomaly detection system
Reduced operational risk
Avoided critical infrastructure downtime
Faster reporting
Streamlined analytics for 24×7 access
“InfluxDB has a very awesome stack, which gave us everything we needed when constructing the system.”